DEIA Spotlight - Kara Johnson
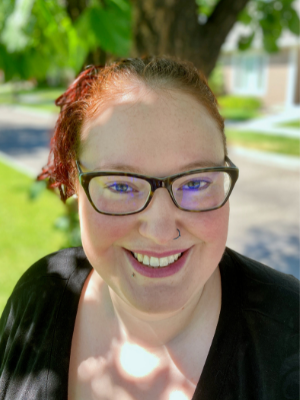
This month we spotlight Kara Johnson, a doctoral candidate in statistics in the Department of Mathematical Sciences. Kara will present a lecture through the Kopriva Science Seminar Series entitled "Promoting Health Behaviors Through Social Influence on Social Networks" at 4:30 p.m. on Tuesday, March 29. Kara's lecture will provide an overview of how her work developing a genetic algorithm is helping public health researchers promote pre-exposure prophylaxis (PrEP) to Black men who have sex with men is a critical tool for preventing HIV transmission. Kara generously took the time to help us get to know her and her work:
What has been your path to working towards your Ph.D. in statistics? In other words, what brought you to this field of study?
My 6th-grade math teacher, Ken Thielman, started me on this path through his support and encouragement. I've loved math ever since taking his class. I started as a math major at MSU, switching to statistics after my first statistics course with Dr. Christa Hayes. I was fascinated with the theory and range of questions statistics can help us answer. I was drawn to my current area of research and motivated to pursue a Ph.D. after learning about Dr. Nicole Carnegie's work controlling for the effect of social interactions when assessing the impact of HIV interventions.
Tell us about your research and how it makes a difference.
I developed my research specifically for the study, using social influence to change opinions about PrEP on networks of Black men who have sex with men. I developed this method because of the lack of available tools. Existing methods were developed for online social networks where data are abundant. However, these methods do not work on the smaller in-person social networks with limited data used in public health and social science research. The intervention and analysis using the genetic algorithm could be applied to change health behaviors related to stigmatized health conditions—such as substance use disorders and sexually transmitted infections—in underserved and marginalized populations. Still, the tool is now available to anyone wanting to model opinion diffusion on small networks with limited data.
Tell us a little bit about what brought you to MSU.
I first came to MSU as an undergrad because of a combination of academic opportunities, scholarships and the culture of MSU and Bozeman. I stayed at MSU because of the welcoming and supportive environment within the Department of Mathematical Sciences.
What are your hopes for after you finish this part of your career?
I'm hoping to pivot slightly toward responsible machine learning (ML) or artificial intelligence (AI), taking either an applied or research approach. This idea is frequently referenced using the acronym FATE (fairness, accountability, transparency and ethics) in AI. While AI has the potential for societal benefit by removing humans from decision-making processes, flaws in implementation—such as training these models on historical data—can result in models that exacerbate societal biases while being presented as objective. For example, Amazon's system for rating candidates was biased against female candidates for software developer or other technical roles and predictive or data-driven policing can result in feedback loops, increasing police presence in already over-policed communities. I can apply my statistical background in sampling biased and modeling assumptions to make a difference in this area. For those interested in an accessible introduction to this issue, I recommend Weapons of Math Destruction by Cathy O'Neil.
Because March is Women's History Month, we would love to hear from you about resources for Women in STEM that you have found helpful.
While specifically related to my research areas, I would like to highlight Women in Network Science (WiNS) and Women in Machine Learning (WiML). WiNS is an organization for women, trans and non-binary gender network scientists which offers a wealth of networking and educational opportunities. The mission of WiML is to increase the number of women in machine learning, help women in machine learning succeed professionally, and increase the impact of women in the machine learning community. I highly recommend the WiML mailing list for opportunities in academia and industry where diversity is valued. For the broader statistics and data science community, the Caucus for Women in Statistics (CWS) and the Women in Statistics and Data Science Conference (WSDS) through the American Statistical Association (ASA) offer excellent resources for women in all stages of their careers.
What do you like to do when you are not in your research/academic mode?
I have varied interests and enjoy crafts, such as cross-stitching and knitting, cooking, PC and board games, and sci-fi and fantasy books, movies and TV.